
Have that fourth residual which is 0.5 squared, 0.5 squared, so once again, we tookĮach of the residuals, which you could view as the distance between the points and what
#Residual statistics plus
Residual which is negative one, so plus negative one squared and then finally, we This blue or this teal color, that's zero, gonna square that. To the second residual right over here, I'll use We're going to take this first residual which is 0.5, and we're going to square it, we're going to add it And so, when your actual isīelow your regression line, you're going to have a negative residual, so this is going to be So, two minus three isĮqual to negative one. Well, when X is equal to two, you have 2.5 times two, which is equal to five Going to be the actual, when X is equal to two is two, minus the predicted. Sits right on the model, the actual is the predicted, when X is two, the actual is three and what was predictedīy the model is three, so the residual here isĮqual to the actual is three and the predicted is three, so it's equal to zero and then last but not least, you have this data point where the residual is So, once again you haveĪ positive residual. Is equal to six minus 5.5 which is equal to 0.5. So, you have six minus 5.5, so here I'll write residual Now, the residual over here you also have the actual pointīeing higher than the model, so this is also going toīe a positive residual and once again, when X is equal to three, the actual Y is six, the predicted Y is 2.5 times three, which is 7.5 minus two which is 5.5. 5, so this residual here, this residual is equal to one minus 0.5 which is equal to 0.5 and it's a positive 0.5 and if the actual point is above the model you're going to have a positive residual. So, for example, and we'veĭone this in other videos, this is all review, the residual here when X is equal to one, we have Y is equal to one but what was predicted by the model is 2.5 times one minus two which is. The linear regression predict for a given X? And this is the actual Y for a given X. Now, when I say Y hat right over here, this just says what would So, just as a bit of review, the ith residual is going toīe equal to the ith Y value for a given X minus the predicted Y value for a given X. So, what we're going to do is look at the residualsįor each of these points and then we're going to find The root-mean-square error and you'll see why it's called this because this really describes We could consider this toīe the standard deviation of the residuals and that's essentially what This case, a linear model and there's several names for it. Going to do in this video is calculate a typical measure of how well the actual data points agree with a model, in This forms an unbiased estimate of the variance of the unobserved errors, and is called the mean squared error.ĭoes p = 0 in this case? Or does p = 1 since y-hat is attempting to estimate the parameter that is the true y at each x? Since this is a biased estimate of the variance of the unobserved errors, the bias is removed by dividing the sum of the squared residuals by df = n − p − 1, instead of n, where df is the number of degrees of freedom ( n minus the number of parameters p being estimated - 1).
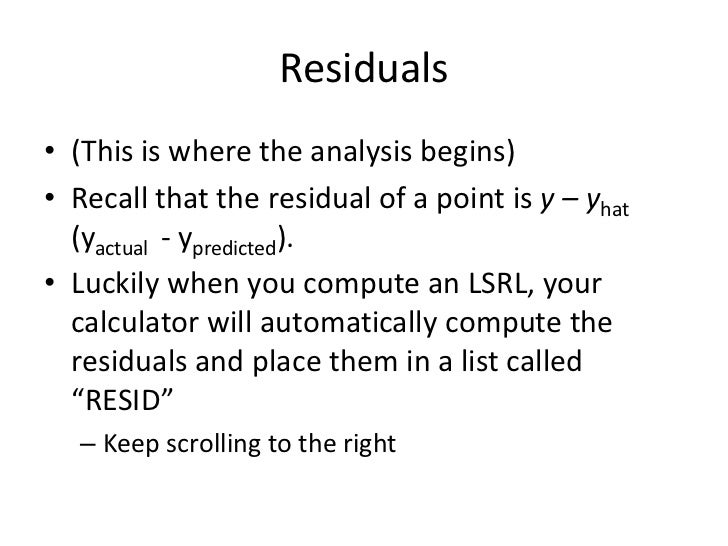
If that sum of squares is divided by n, the number of observations, the result is the mean of the squared residuals. The mean squared error of a regression is a number computed from the sum of squares of the computed residuals, and not of the unobservable errors. However, a terminological difference arises in the expression mean squared error (MSE). If one runs a regression on some data, then the deviations of the dependent variable observations from the fitted function are the residuals. Given an unobservable function that relates the independent variable to the dependent variable – say, a line – the deviations of the dependent variable observations from this function are the unobservable errors. In regression analysis, the distinction between errors and residuals is subtle and important, and leads to the concept of studentized residuals.

I'm not entirely convinced of my attempted explanation since the Wikipedia article on errors/residuals has this tidbit: Is the difference because here we are only looking at error variance due to intercept only (hence + and - error lines that are 'shifted' above and below the regression line) rather than slope and intercept (which would include + and - 'shifts' as well as 'rotation' around the coordinate (x-bar, y-bar))? 4:20, Sal notes that we should divide by (n-1), but I've seen elsewhere (n-2) in the denominator since y-hat estimation costs us two degrees of freedom - one for the intercept term and one for the slope term of our regression line.
